在当今数据驱动的世界中,数据库管理系统(DBMS)扮演着至关重要的角色,它们不仅存储和管理数据,还提供了强大的查询和分析工具,帮助组织从大量数据中提取有价值的信息,MySQL 5作为一个广泛使用的开源关系型数据库管理系统,以其稳定性、可靠性和易用性而闻名,本文将深入探讨MySQL 5数据库的使用,特别是通过一个Caffe分类范例来展示如何利用MySQL进行数据处理和分析。
一、MySQL 5数据库简介
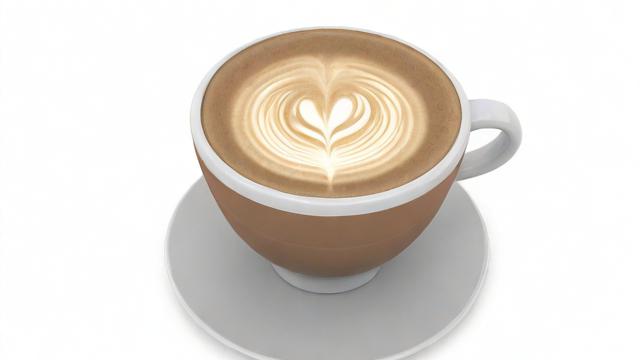
MySQL是一个由瑞典MySQL AB公司开发的关系型数据库管理系统,后被Sun Microsystems收购,最终成为Oracle公司的一部分,MySQL使用结构化查询语言(SQL)作为其数据库访问和操作的标准语言,MySQL 5是该系列的一个主要版本,引入了许多新特性,如存储过程、触发器、视图等,极大地增强了其功能和灵活性。
二、Caffe分类范例
Caffe是一个深度学习框架,广泛用于图像识别、语音识别和其他机器学习任务,在这个范例中,我们将使用Caffe对一组图像进行分类,并将结果存储在MySQL数据库中进行分析,这个流程包括以下几个步骤:
1、数据准备:收集并预处理待分类的图像数据集。
2、模型训练:使用Caffe框架训练一个图像分类模型。
3、模型部署:将训练好的模型部署到生产环境中。
4、结果存储:将分类结果存储到MySQL数据库中。
5、数据分析:从MySQL数据库中检索数据并进行分析。
三、详细步骤与代码示例
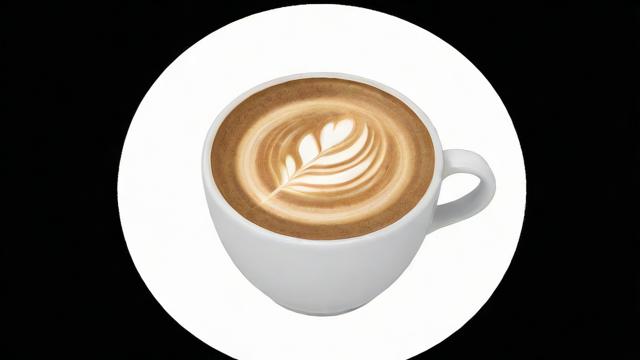
1. 数据准备
我们需要准备用于训练的图像数据集,这通常涉及收集图像、标注类别以及进行必要的预处理,如缩放、裁剪等。
import os from PIL import Image def preprocess_images(input_dir, output_dir): if not os.path.exists(output_dir): os.makedirs(output_dir) for filename in os.listdir(input_dir): if filename.endswith('.jpg'): img = Image.open(os.path.join(input_dir, filename)) img = img.resize((224, 224)) # Resize to Caffe's expected input size img.save(os.path.join(output_dir, filename)) preprocess_images('/path/to/input', '/path/to/output')
2. 模型训练
我们使用Caffe框架训练一个图像分类模型,这里假设我们已经有一个预训练的模型作为起点。
Assuming we have a pre-trained model and solver files caffe train -solver /path/to/solver.prototxt
3. 模型部署
一旦模型训练完成,我们可以将其部署到生产环境中进行实时分类。
import caffe import numpy as np from PIL import Image model_def = '/path/to/deploy.prototxt' model_weights = '/path/to/model.caffemodel' net = caffe.Net(model_def, model_weights, caffe.TEST) def classify_image(image_path): img = Image.open(image_path) img = img.resize((224, 224)) img_data = np.array(img, dtype=np.float32) img_data = img_data.transpose((2, 0, 1)) # HWC to CHW img_data = img_data[np.newaxis, :] # Add batch dimension net.blobs['data'].data[...] = img_data output = net.forward() return output Example usage print(classify_image('/path/to/image.jpg'))
4. 结果存储
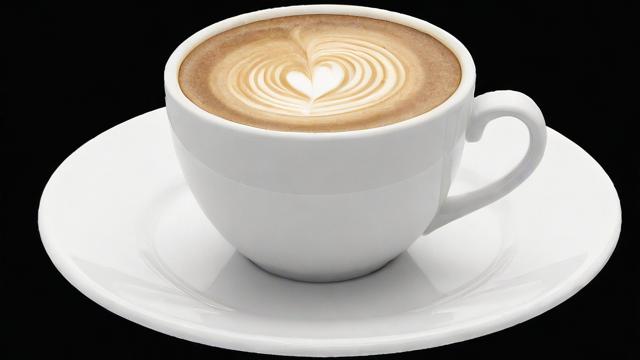
我们将分类结果存储在MySQL数据库中,为此,我们需要先创建一个数据库和相应的表结构。
CREATE DATABASE image_classifier; USE image_classifier; CREATE TABLE classification_results ( id INT AUTO_INCREMENT PRIMARY KEY, image_path VARCHAR(255), category VARCHAR(255), confidence FLOAT, created_at TIMESTAMP DEFAULT CURRENT_TIMESTAMP );
我们可以编写Python代码将分类结果插入到数据库中。
import pymysql import datetime def insert_classification_result(image_path, category, confidence): connection = pymysql.connect(host='localhost', user='root', password='password', db='image_classifier') try: with connection.cursor() as cursor: sql = "INSERT INTO classification_results (image_path, category, confidence) VALUES (%s, %s, %s)" cursor.execute(sql, (image_path, category, confidence)) connection.commit() finally: connection.close() Example usage insert_classification_result('/path/to/image.jpg', 'category', 0.95)
5. 数据分析
我们从MySQL数据库中检索数据并进行分析,这可能包括计算准确率、混淆矩阵等指标。
import pymysql import pandas as pd def fetch_classification_results(): connection = pymysql.connect(host='localhost', user='root', password='password', db='image_classifier') try: with connection.cursor() as cursor: sql = "SELECT * FROM classification_results" cursor.execute(sql) results = cursor.fetchall() df = pd.DataFrame(results, columns=['id', 'image_path', 'category', 'confidence', 'created_at']) return df finally: connection.close() Example usage df = fetch_classification_results() print(df.head())
四、相关问答FAQs
Q1: 如何在MySQL中优化查询性能?
A1: 在MySQL中优化查询性能可以从多个方面入手,包括但不限于以下几点:使用索引加速查询、避免全表扫描、合理设计数据库架构、使用合适的数据类型、定期维护数据库(如清理碎片、更新统计信息等)、以及编写高效的SQL语句,还可以考虑使用缓存机制或读写分离等策略来进一步提升性能。
Q2: Caffe框架在图像分类中的优势是什么?
A2: Caffe框架在图像分类中具有多方面的优势,它支持多种类型的神经网络层,使得模型构建更加灵活多样,Caffe提供了丰富的预训练模型和网络结构,可以大大缩短模型训练的时间和成本,Caffe还具有良好的扩展性和可移植性,可以在多种硬件平台上运行,Caffe的社区非常活跃,提供了丰富的资源和支持,有助于解决在使用过程中遇到的问题。
以上内容就是解答有关“mysql 5 数据库 范例_Caffe分类范例”的详细内容了,我相信这篇文章可以为您解决一些疑惑,有任何问题欢迎留言反馈,谢谢阅读。